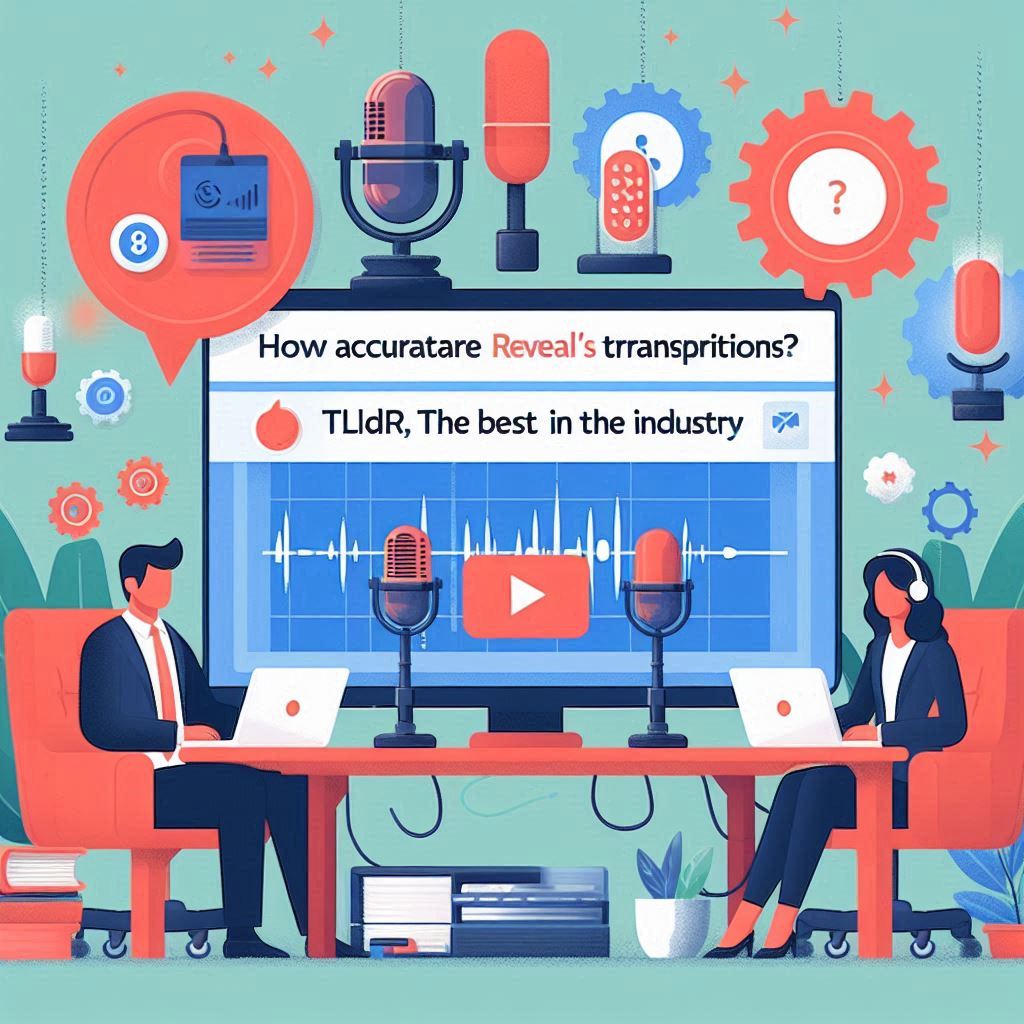
When it comes to converting spoken language into written text, the accuracy and quality of transcription are crucial. Various AI based transcription tools are available in the market, each with different levels of performance. This blog post will break down key metrics that determine the quality of these transcriptions, focusing on a recent benchmark study.
Key Metrics for Transcription Quality
Word Accuracy Rate
Definition: This metric measures how accurately a transcription model can convert spoken words into written text.
Performance: Reveal correctly transcribes a higher percentage of words compared to competitors like OpenAI, Microsoft, and Google.
Language | Reveal | OpenAI | Microsoft | |
---|---|---|---|---|
English | 92.7% | 91.6% | 90.6% | 86.4% |
Spanish | 95.2% | 94.0% | 92.8% | 91.3% |
German | 92.5% | 91.6% | 91.1% | 87.3% |
Word Error Rate (WER)
Definition: WER calculates the errors made in transcriptions, including insertions, deletions, and substitutions of words.
Performance: Lower WER indicates better transcription quality. Reveal’s model has a WER of 7.3% in English, 4.8% in Spanish, and 7.5% in German, outperforming other models which have higher error rates.
Language | Reveal | OpenAI | Microsoft | |
---|---|---|---|---|
English | 7.3% | 8.4% | 9.4% | 13.6% |
Spanish | 4.8% | 6.0% | 7.2% | 8.7% |
German | 7.5% | 8.4% | 8.9% | 12.7% |
Consecutive Error Types
Definition: This metric looks at specific types of errors over a long period, such as fabrications (incorrectly added words), omissions (missing words), and hallucinations (strings of consecutive errors).
Performance: Reveal shows a 30% reduction in hallucination rates compared to Whisper Large-v3, with lower rates of fabrications (5.2% vs. 8.8%) and omissions (5.6% vs. 7.1%).
Error Type | Reveal | Whisper Large-v3 |
---|---|---|
Fabrications | 5.2% | 8.8% |
Omissions | 5.6% | 7.1% |
Hallucinations | 12.9% | 18.4% |
Importance of Accurate Transcriptions
Accurate transcriptions are vital for various applications, including:
- Summaries: Creating accurate summaries of meetings, interviews, and conferences.
- Customer Insights: Analyzing customer calls to gather insights and improve service.
- Metadata Tagging: Adding accurate tags to audio content for better search and organization.
- Qualitative Research: Synthesizing qualitative research data, such as user and market research, to derive meaningful insights.
How Benchmarks Are Conducted
The benchmark study used over 250 hours of audio data from various sources, including public datasets and in-house recordings. These datasets cover a wide range of speech types, including phone calls, podcasts, and broadcasts. By testing different models on these datasets, the study ensured a comprehensive evaluation of each model’s performance.