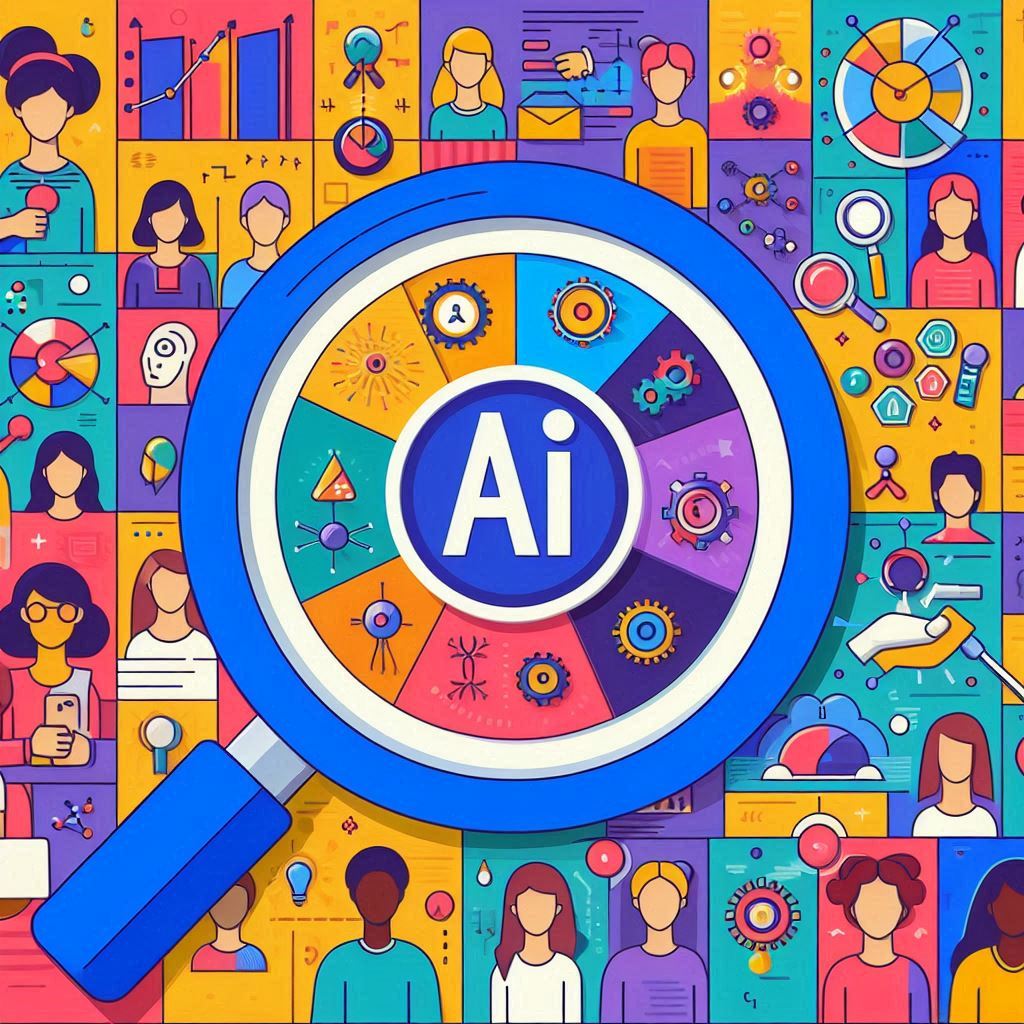
Artificial Intelligence (AI) is advancing at an unprecedented pace, reshaping industries in ways we could scarcely imagine just a few years ago. Qualitative research—historically reliant on human intuition, interpretation, and time-intensive methodologies—is now poised for a transformation. AI’s increasing intelligence and rapidly decreasing costs are making it more accessible and applicable than ever before. The question is no longer if AI will influence qualitative research but how it will redefine the field.
The Accelerating Pace of AI Development
AI is not evolving linearly—it is advancing exponentially. OpenAI’s CEO Sam Altman has underscored this rapid trajectory, stating, "The cost of using AI will decrease by a factor of ten every year. (source)" This phenomenon, coupled with continuous improvements in AI’s cognitive capabilities, means that cutting-edge AI models, once accessible only to tech giants, are now available to a broader range of industries—including qualitative research.
Sam has also highlighted AI’s accelerating proficiency in programming, noting that AI has gone from being the equivalent of the millionth-best programmer to being ranked among the top 50. He anticipates that by the end of 2025, AI will be the world’s best programmer. This rapid improvement suggests that AI’s capabilities in qualitative research will similarly advance, making it an increasingly indispensable tool for researchers.
The implications of this rapid evolution are profound. AI-driven tools are not just making qualitative research more efficient; they are fundamentally altering the nature of research itself, shifting the focus from manual data handling to strategic insight extraction.
AI’s Expanding Role in Qualitative Research
The impact of AI on qualitative research is multi-faceted. It doesn’t just speed up existing workflows—it fundamentally changes how research is conducted, analyzed, and acted upon. Here’s how:
1. Enhanced Data Collection and Analysis
Historically, qualitative research has involved lengthy processes: recording interviews, manually transcribing data, coding themes, and synthesizing insights. These steps have traditionally taken weeks, if not months. AI drastically accelerates this by automating these processes with higher accuracy and at a fraction of the cost.
AI-powered tools can transcribe interviews, extract key themes, and generate summaries within minutes. Natural Language Processing (NLP) algorithms are increasingly adept at understanding human emotions, sentiment, and even sarcasm—elements that were once thought to be uniquely human competencies.
For example, AI-led interviews have been found to elicit richer responses. A A study from the London School of Economics revealed that respondents in AI-conducted interviews provided 142% more words per response compared to traditional human-led interviews. This suggests that AI, when properly designed, can help researchers unlock deeper and more candid insights from participants.
2. The Rise of the 10x Researcher
Just as AI has enabled 10x engineers—developers whose productivity is amplified by AI-powered coding assistants—AI is also creating the 10x researcher. A 10x researcher is someone who can leverage AI to:
-
Process and analyze qualitative data at scale
-
Surface deeper insights faster
-
Automate tedious tasks and focus on high-value interpretation
-
Visualize complex themes dynamically instead of manually coding transcripts
This shift is not just about efficiency but about capability expansion. A single researcher, empowered with AI, can now conduct large-scale qualitative studies that previously required entire teams. They can explore broader themes, identify emerging trends faster, and generate insights that would have been impossible to surface with traditional methods.
3. AI-Augmented Research Workflows: Before vs. After
To illustrate AI’s impact, let’s compare the traditional research workflow with an AI-powered workflow:
Before AI:
-
Researchers conduct and record interviews manually.
-
Hours are spent transcribing conversations.
-
Analysts manually code qualitative data, limiting sample sizes due to resource constraints.
-
Insights take weeks or months to surface.
After AI:
-
AI automates transcription in real-time, reducing turnaround times from hours to minutes.
-
NLP models extract key themes and sentiments immediately.
-
Researchers can analyze thousands of responses simultaneously, instead of being limited by manpower.
-
Insights are surfaced in real-time, allowing for more agile decision-making.
4. More Robust Pattern Recognition
Human researchers are skilled at identifying patterns in qualitative data, but they are also prone to cognitive bias, fatigue, and constraints in handling large datasets. AI, on the other hand, can process millions of qualitative data points, recognizing patterns and connections that might be overlooked in manual analysis. Advanced machine learning algorithms can:
-
Detect emerging trends before they become obvious.
-
Identify subtle correlations between themes across datasets.
-
Provide a more objective, bias-reduced perspective on qualitative insights.
Preparing for an AI-Integrated Future
1. Understanding the Role of AI in Research
As AI transforms qualitative research, professionals must develop a clear understanding of what AI can and cannot do. While AI excels at pattern recognition, summarization, and speed, human researchers remain essential for:
-
Contextualizing findings: AI can surface themes, but researchers must interpret their implications.
-
Asking the right questions: AI can analyze responses, but it lacks the ability to strategically frame research questions.
-
Ensuring ethical integrity: AI-driven insights must be examined for bias, fairness, and ethical considerations.
2. Developing AI Literacy
To stay ahead, qualitative researchers must become proficient in AI tools and methodologies. This means:
-
Learning how AI-driven transcription, sentiment analysis, and NLP models work.
-
Experimenting with AI tools to understand their strengths and weaknesses.
-
Staying informed on emerging AI research and best practices.
3. Shifting Toward Higher-Order Thinking
With AI handling much of the manual work, qualitative researchers will need to focus on:
-
Strategic insight generation: Moving beyond data collection to storytelling and strategic decision-making.
-
Synthesizing cross-disciplinary knowledge: AI will enable broader data integration, requiring researchers to connect qualitative insights with quantitative and behavioral data.
-
Developing new AI-driven methodologies: Rather than simply applying AI to old workflows, forward-thinking researchers will innovate new methods enabled by AI capabilities.
4. Addressing Ethical Considerations
AI introduces new ethical dilemmas in qualitative research. Issues surrounding data privacy, algorithmic bias, and consent must be carefully managed. Researchers should proactively establish guidelines for responsible AI usage, ensuring transparency in how AI processes and interprets qualitative data.
The Future of AI in Qualitative Research
The convergence of AI and qualitative research is not just a passing trend—it’s an evolution that will redefine the industry. Researchers who embrace AI’s capabilities will find themselves equipped with more powerful tools, greater efficiency, and deeper insights than ever before.
The future is not AI versus researchers; it’s AI empowering researchers to become 10x more effective, unlocking richer, more meaningful insights at a scale and speed never before possible.
Further Reading & References
-
Sam Altman on AI Cost Reduction: Business Insider
-
AI in Qualitative Research: London School of Economics
-
Ethical Considerations in AI Research: Inside Higher Ed
-
AI and the Future of Research Methodologies: Nesta