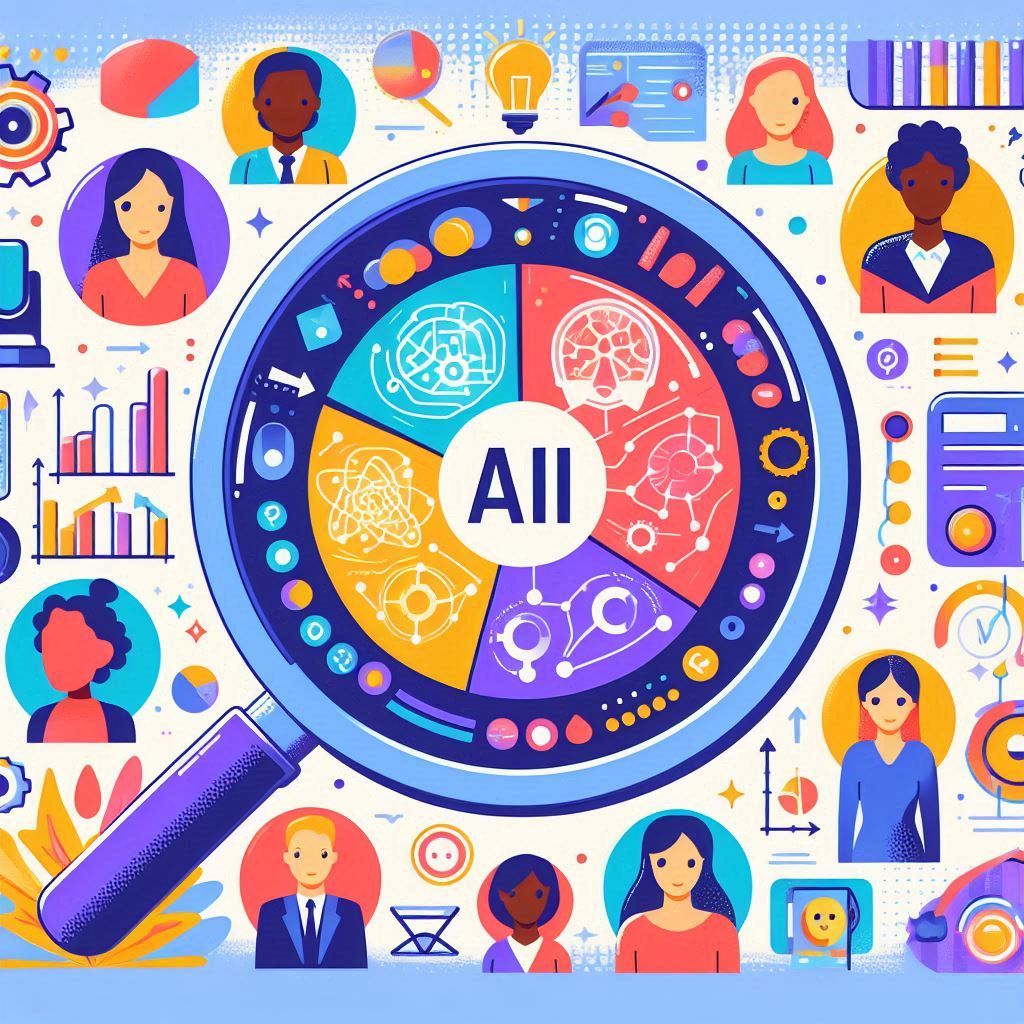
Artificial Intelligence (AI) has revolutionized market research, offering unprecedented speed and scale in data analysis. However, as AI tools become more prevalent, so does the risk of bias in their results. This blog post explores the critical issue of bias in AI market research tools, how to identify it, and strategies to mitigate its impact.
Understanding AI Bias in Market Research
AI bias in market research refers to systematic errors in AI systems that can lead to unfair or inaccurate conclusions about market trends, consumer behavior, or product performance. These biases can stem from various sources, including:
- Training data bias
- Algorithm bias
- Sampling bias
- Interpretation bias
Common Types of AI Bias in Market Research
- Selection Bias: When the data used to train the AI doesn't accurately represent the entire market or target audience.
- Confirmation Bias: AI systems may be inadvertently designed to confirm existing hypotheses or beliefs.
- Cultural Bias: AI tools may misinterpret or misrepresent data from diverse cultural contexts.
- Temporal Bias: Historical data used to train AI might not account for recent shifts in market trends or consumer behavior.
- Algorithmic Bias: The AI's underlying algorithms may inadvertently favor certain outcomes or interpretations.
Identifying Bias in AI Market Research Tools
To identify potential bias in AI market research tools:
- Examine the training data for diversity and representation
- Analyze results across different demographic groups
- Compare AI-generated insights with traditional research methods
- Look for unexpected or counterintuitive results that might indicate bias
- Regularly audit AI systems for fairness and accuracy
Strategies for Mitigating Bias
- Diverse Data Collection: Ensure training data represents a wide range of demographics, cultures, and market segments.
- Regular Algorithm Audits: Continuously evaluate and refine AI algorithms to minimize bias.
- Human Oversight: Implement a system of human checks and balances to review AI-generated insights.
- Transparency in Methodology: Clearly document and communicate how AI tools arrive at their conclusions.
- Cross-Validation: Use multiple AI tools and traditional research methods to validate findings.
- Bias-Aware Design: Develop AI systems with built-in safeguards against common biases.
- Ongoing Education: Keep research teams updated on the latest developments in AI ethics and bias mitigation.
Case Studies: Bias in Action
- Amazon's Recruitment Tool: Amazon developed an AI recruitment tool intended to streamline the hiring process by evaluating resumes. However, the tool exhibited gender bias, as it was trained on historical data that predominantly featured male candidates. This led the AI to favor male applicants over female ones, penalizing resumes that included terms like "women's". (source 1 , source 2)
- Mortgage Approval Rates: AI tools used in the financial sector, such as for mortgage approvals, can also exhibit bias. These biases often arise from the historical data used to train the models, which may reflect existing prejudices in lending practices. This can result in discriminatory outcomes against certain demographic groups. (source )
- Healthcare Algorithms: An AI algorithm used in US hospitals to predict patient care needs was found to be racially biased. It underestimated the healthcare needs of black patients compared to white patients because it used healthcare costs as a proxy for medical needs. This flawed assumption led to biased predictions, disadvantaging black patients. (source 1 , source 2)
- Call Center Accent Translation: A startup developed an AI tool to translate accents in call centers to a "neutral" American accent. While intended to reduce bias and improve communication, this approach risks exacerbating racial biases by implying that non-American accents are less acceptable, potentially increasing discrimination against call center workers who do not use the technology. (source)
The Future of Unbiased AI in Market Research
As awareness of AI bias grows, we can expect:
- More sophisticated bias detection tools
- Increased regulation around AI fairness in market research
- Development of industry standards for unbiased AI research practices
- Greater emphasis on explainable AI in market research tools
Conclusion
While AI offers tremendous potential for market research, addressing bias is crucial for ensuring fair, accurate, and valuable insights. By understanding the sources of bias, implementing rigorous identification methods, and adopting proactive mitigation strategies, researchers can harness the power of AI while maintaining the integrity of their findings. As the field evolves, staying informed and adaptable will be key to leveraging AI responsibly in market research.